Data quality remains the critical bottleneck in deploying highly accurate generative AI systems. Gartner predicts that 30% of GenAI initiatives will fail due to data challenges, illustrating how critical it is for enterprises to develop strategies that ensure high-quality data at scale.
The increasing complexity of AI use cases compounds these obstacles. Traditional annotation platforms struggle to keep up, offering only a fixed set of single-use-case editors that no longer fit modern AI projects' diverse and evolving needs. SuperAnnotate enables customers to bring innovative AI products to market faster through its unique annotation application Builder and leading workforce management.
SuperAnnotate Integrating NVIDIA NeMo Evaluator
Today, SuperAnnotate is announcing a technical collaboration to integrate NVIDIA NeMo Evaluator, an enterprise-grade microservice that provides industry-standard benchmarking of generative AI models, synthetic data generation, and end-to-end retrieval-augmented generation (RAG) pipelines, into its platform. NeMo Evaluator provides simple API endpoints and enables customers to leverage large language models (LLMs) as automated judges, eliminating the need for complex custom evaluation code, and to run inference at scale.
This integration enables organizations to easily incorporate both human expertise and LLM as judges in their data pipelines, finding the optimal balance between costs and accuracy for their AI projects.
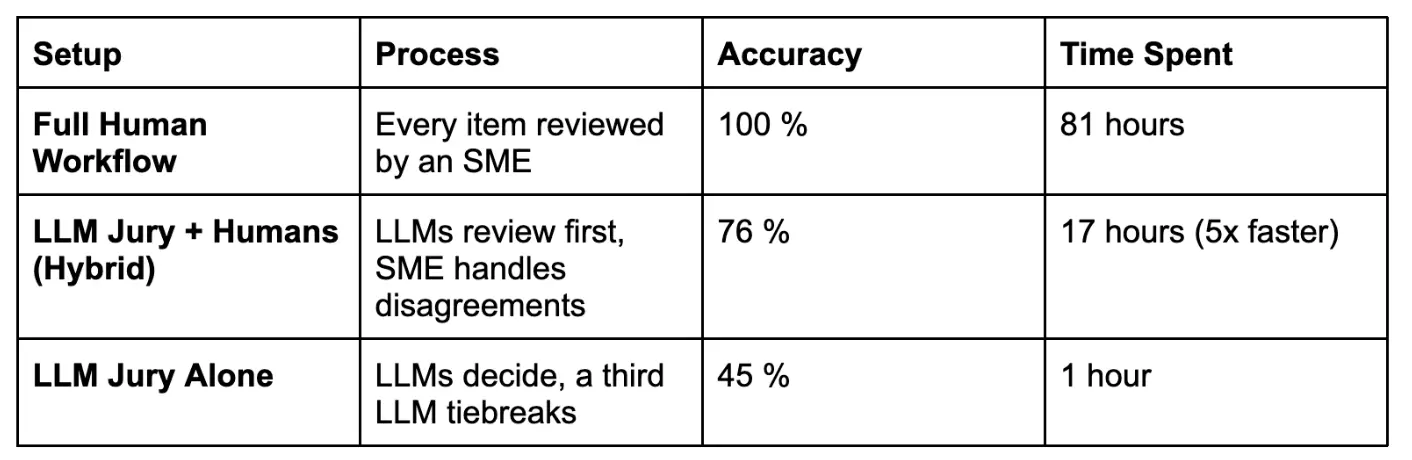
Case Study:
We tested this combined approach with a large enterprise customer, evaluating 148 chat conversation examples—a dataset that historically required 81 hours of purely manual evaluation.
Given the high volume of tasks—8,000–10,000 items per week—the customer sought a more efficient approach. We integrated NeMo Evaluator’s LLM-as-a-Judge into an AI/human hybrid collaboration workflow built in SuperAnnotate to assess how (LLM) this workflow impacts time and accuracy.
The Annotation Process
To test the accuracy and potential time savings of using this approach, we created an experimental setup comparing this hybrid approach to a fully human and automated evaluation system. We used OpenAI’s GPT-4o and Meta’s llama-3.3-70b-instruct in the experiment as the LLM Jury. Each LLM received a prompt containing the same instructions and guidelines that subject matter experts (SMEs) use.
The labeling task required selecting the “winner” (A, B, or Tie) in each chat based on the workflow below.
- LLM Jury: Each LLM conducts an independent evaluation
- Consensus Check: If all LLMs agree, the annotation is automatically accepted.
- Escalation to SME: If the models disagree, the item is flagged for expert review.
These results highlight a significant discrepancy in accuracy between human workflows and automated setups. Compared to human workflows, the hybrid approach reduces time more than it reduces quality, making it a viable option for balancing cost, speed, and accuracy based on the project's quality requirements and risk tolerance. Additionally, it shows that integrating human experts into an automated workflow can significantly enhance evaluation quality, leading to more reliable downstream models.
Key takeaways:
- Human evaluation offers greater accuracy but at the highest cost.
- LLM Jury setups significantly accelerate the process but at the cost of reduced accuracy.
- The hybrid approach suggests it can be effectively calibrated to balance speed, cost, and quality for a given project if accuracy is not paramount.
Looking Ahead
For any enterprise struggling with scaling generative AI, particularly around data quality and model evaluation, combining AI-driven and human evaluation offers a proven way to shorten labeling cycles, reduce cost, and achieve high data quality. Organizations can build more reliable AI pipelines faster by leveraging the SuperAnnotate platform for annotation with NVIDIA NeMo Evaluator.
Enterprises looking to scale generative AI while maintaining high data quality can benefit from a flexible approach that allows for AI-driven and human evaluation. By using SuperAnnotate’s annotation platform integrated with NVIDIA NeMo Evaluator, organizations can streamline labeling, reduce costs, and build more reliable AI pipelines—faster and more efficiently.
Ready to transform your model evaluation with SuperAnnotate accelerated by NVIDIA?
