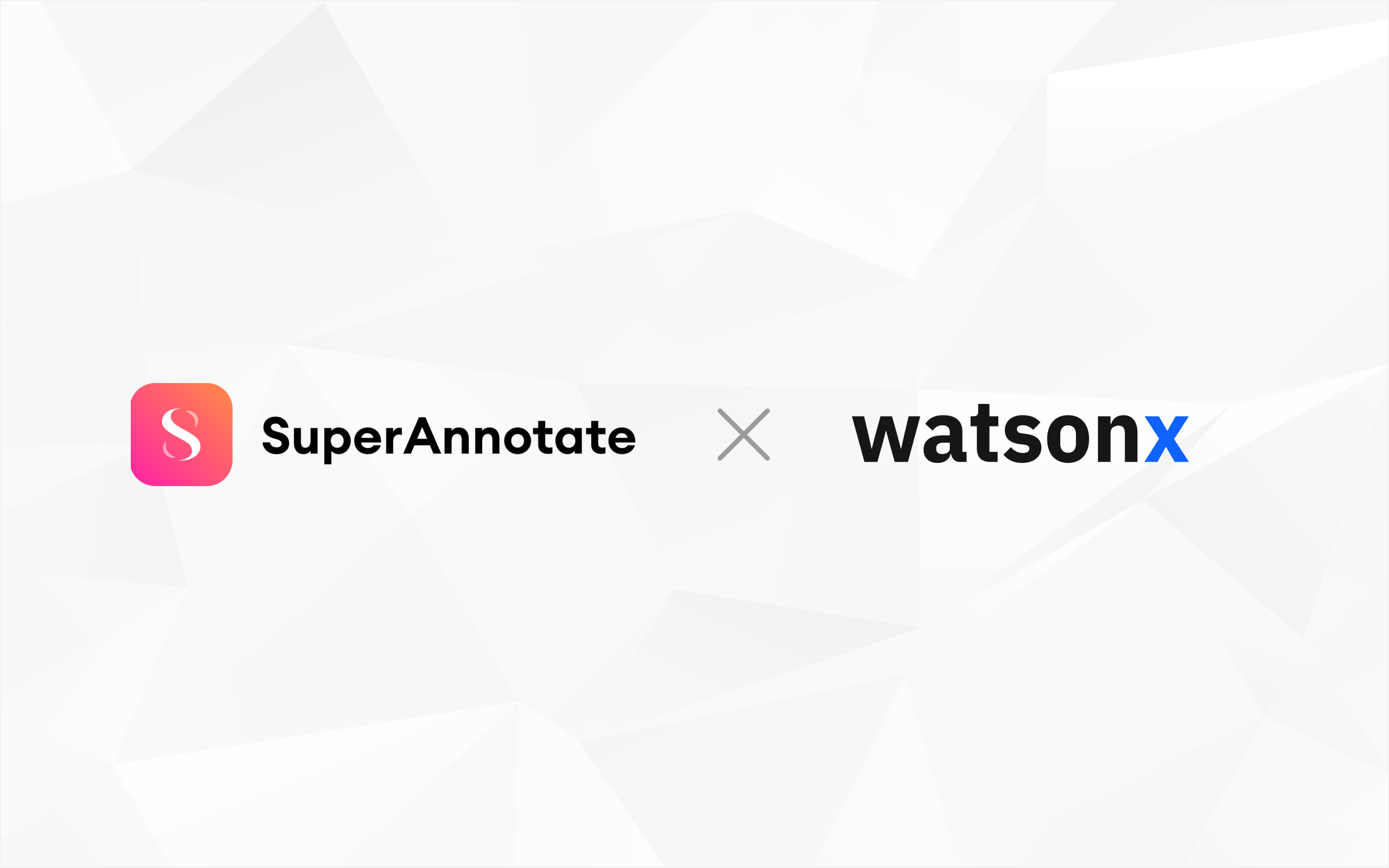
Large language models (LLMs) are becoming crucial for businesses seeking to extract more value from their data. By adapting these models to specific use cases and evaluating their performance, companies can ensure the models meet their unique needs. This adaptation allows businesses to direct the models towards desired outcomes efficiently, aligning with particular use cases. Evaluating these models is also essential, as it helps companies make informed decisions about their deployment and identify areas for improvement.
SuperAnnotate and IBM are now partnering to make the path to deploying prompt-tuned LLMs easier. In this blog post, we'll explore the ins and outs of this partnership and show how you can start using these tools in your operations.
SuperAnnotate x IBM watsonx integration
Today, a few hurdles make LLM fine-tuning unnecessarily complicated—tools for fine-tuning often assume that you have a dataset ready to go that perfectly captures how the model should behave and have limited tooling to evaluate model performance thoroughly. This unnecessarily complicates training infrastructure, leaving users to build integrations between various tools or build their tools from scratch.
Another problem with traditional fine-tuning is that it requires a lot of data and resources. You need to gather and label new examples each time you want to adapt the model to a specific task. This process is time-consuming and costly, especially as models grow larger.
The partnership between IBM and SuperAnnotate aims to make it easier and quicker for companies to work with large language models. We focus on simplifying the creation and improvement of datasets and the evaluation of model performance, helping streamline the entire process of model integration and data transfer.
Model integration
Easily connect SuperAnnotate to models deployed in your watsonx instance to:
- Evaluate LLMs with custom metrics: The setup ensures you can evaluate models or larger systems like retrieval augmented generation (RAG). You can fully customize the setup and integrate it with any system, making the evaluation process more flexible.
- Gather data for LLM fine-tuning: Integrate the models you want to fine-tune into SuperAnnotate, not writing answers from scratch but rather having a model-in-the-loop to help provide answers.
Data integration
Seamlessly move data between SuperAnnotate and watsonx using:
- Automated data export for prompt-tuning: Easily configure data export to watsonx from SuperAnnotate in a format ready for prompt-tuning – a technique that allows adopting the model to specialized tasks by using specific cues to guide the model without the need for extensive retraining, which would be the case for traditional fine-tuning.
- Secure data integration: SuperAnnotate can connect directly to your IBM data storage, ensuring that all data at rest remains within your environment.
How does it work?
Here's a short description of how enterprises can use IBM watsonx and SuperAnnotate to gather data for evaluating and tuning their custom LLMs:
- Use IBM's PromptLab to play around with models and get an initial idea of what might work for you.
- If none of the models meet your needs, easily configure a project in SuperAnnotate to gather more data.
- Easily export the data from SuperAnnotate to IBM and start tuning.
- Deploy the models and set up an Evaluation project in SuperAnnotate.
Closing remarks
In conclusion, the partnership between SuperAnnotate and IBM watsonx is set to make it easier for businesses to use large language models effectively. This collaboration simplifies how companies adapt and evaluate these models to meet their specific needs.
With SuperAnnotate's evaluation platform and IBM's advanced data processing, businesses are better equipped to handle challenges with their language models. This partnership provides a straightforward solution for companies looking to remain competitive by leveraging the latest in language model technology, ensuring their models are effective and specifically tailored to their needs.