Building ML models and overseeing data annotation since 2018, we at SuperAnnotate have been helping thousands of Machine Learning teams in all possible industries and with the most unorthodox use-cases. To our surprise, however, a lot of leading ML practitioners struggle with scaling their efforts falling into quite the same traps. Because data is the source code for ML, it’s absolutely critical to address the dataset creation with a high level of granularity and responsibility. As one of the top 100 AI companies in the world by CB insights, we know the effort it takes to get quality data, proper labeling, and a reliable model as a result. That’s why we bring our experience to the roundtable with a series of webinars.

We’ve covered Active learning in-depth on our first webinar, so if this is something you’re interested in, you’re welcome to audit the webinar for free. In our second session, Jason Liang - the co-founder and VP of Business Development at SuperAnnotate - shared his experience at building a pipeline that provides the highest data quality, efficient development cycles, is repeatable and reliable for the future, and provides the most value to your team.
Below, we’ll introduce a short sneak peek of the suggested ideas unveiling the most common mistakes of building an annotation pipeline that grows as you do.
Providing vague instructions to annotators
First of all, annotators can’t do without instructions, and the more detailed they are, the better. Accordingly, make sure to design an instructions document that shares the desired outcome, provides annotation examples with edge cases, and covers the most common ambiguities. It’s easy to mistakenly assume that because annotators know their job, it would be no trouble getting the result you wanted. However, as you scale, the amount of projects increases, so please remember that each of them is industry-specific and demands close attention to detail that one may not have encountered before.
Overlooking project implementation
Once your instructions are ready, communicate those to the team and follow the implementation process. Annotation is not something that has to happen in isolation. You should have a chance to track the team performance and get updated on the progress. Our experience shows that this is one of the most common pitfalls, that’s why we allocate representatives to set up the smoothest communication and ensure our customers receive exactly what they need.
Using homegrown or open-source annotation tools
Don’t get us wrong – using open-source tools for data annotation is totally fine, at first. However, later, as you begin to grow and bring in diverse projects, it will simply lack the flexibility and efficiency to handle multiple workflows. A couple of essential aspects to consider are communication with the team inside the platform, QA, automation and ML features, pipeline integrations, and more.
Coming up with the wrong partner
This is probably the most common and time-consuming issue our clients bump into. Let’s face it – you spend resources, time, and money to research and find a solution that then simply doesn’t fit. How frustrating should that be? We get that, so here’s a piece of advice you may use:
- Take your time to research the software expertise.
- Talk to customer success and see who you trust.
- Rather than thinking about initial costs, focus on the ROI and the ultimate value you’ll get.
In our next webinar, we’ll wrap up the progress in computer vision in 2021 and address opportunities for 2022. Make sure to sign up, save your seat and ensure you get a recording. Click on the banner below to register.
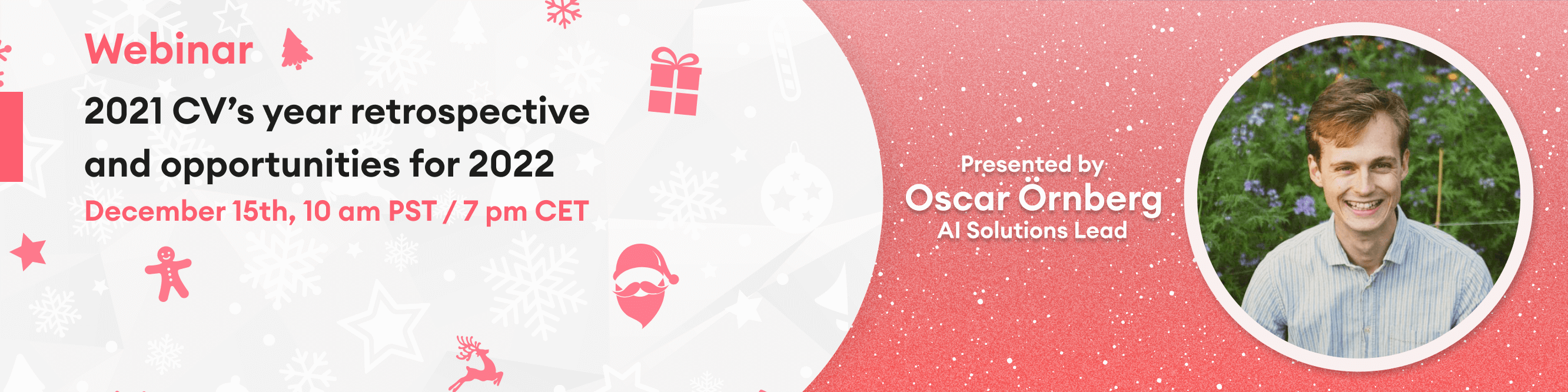
About SuperAnnotate
SuperAnnotate is the world’s leading platform for building the highest quality datasets for computer vision and NLP. With SuperAnnotate, customers create better performing models in less time all while streamlining their ML pipelines. With advanced tooling and QA, ML and automation features, data curation, robust SDK, offline access, and integrated annotation services, SuperAnnotate lets ML teams build incredibly accurate datasets 3-5x faster.
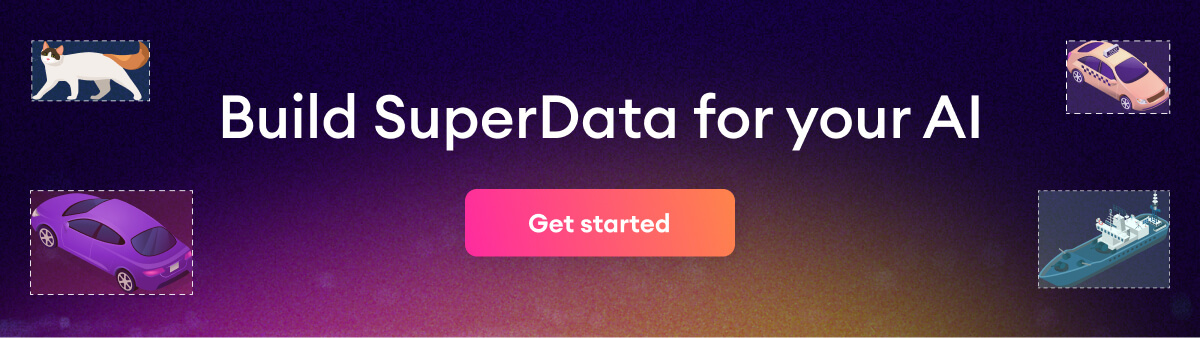