While having humans-in-the-loop execute data annotation tasks seems tempting, these days, companies are inclined to outsource data annotation services to independent vendors. Some of you might, fairly enough, argue that those preferring in-house annotation are merely being cautious about their data security, but we’re here to propose an alternative perspective.
Odds are you will experience the urgent need for crowdsourced data for training your machine learning (ML) model as your project expands in scale. We hope this post helps you make informed decisions before that happens. Let’s find out why exactly outsourcing a data annotation project is the right choice for you.
- Why outsource your data annotation project
- Precautions when choosing the right vendor
- Don’t be afraid to negotiate your package
- Find your service provider match with SuperAnnotate
- Final thoughts
Why outsource your data annotation project
As obscure as it may seem, outsourcing a data annotation project has a myriad of advantages and is likely to boost your computer vision (CV) pipeline forward if implemented in the right place at the right time.

Foolproof security
Let’s set this clear right off the bat before you have a fountain of questions in your head: security can be both a concern and a motivation behind your choice whether to outsource or not. Opinions do split when it comes to trusting a third party. That’s especially the case with companies that consider outsourcing sensitive data, who might be reluctant to share confidential information, let alone deal with the data leakage.
However, professional outsourcing companies with years of experience behind their shoulders have publicly available ethics and integrity guidelines, which you may want to check out before purchasing their services.
Mitigating internal bias
In-house lenient companies are at the risk of acquiring internal bias without necessarily being aware of it. That happens when annotators have a predefined understanding of the way the algorithms function, which also substantiates the existence of a prejudiced and faulty outcome towards the end.
ML training data is subject to the influence of three major bias causes:
- Sample bias: when the data that will later serve for training a model is not in coherence with the environment.
- Prejudiced bias: when the training data is impacted by gender, cultural and other stereotypes.
- Internal bias: when annotation teams have prior expectations as to how the model performs in general.
Outsourcing your data annotation project contributes to mitigating the bias causes to the greatest extent possible.
Better quality training datasets
Continuous polishing of training data quality is one of the least enjoyable chores in ML, but outsourcing to the right company does pay off the money and effort put into finding your service match. Feel free to refer to our client feedback for more.
With the right vendor, the annotation quality won’t be a concern as you’ll have time to spruce other components of your CV pipeline while experts deliver quality results.
Fast service delivery
Time is an indisputable incentive when it comes to project annotation. Better AI unlocks a better world, yet there are countless companies and individuals running the same race as you to own the keys for that world. It would be smart to calculate the opportunity cost, the loss or gain of your decision before you go in-house or move ahead with data annotation outsourcing. The timely availability is always there as a major benefit; the way you opt to utilize that time determines the further success of your CV project.
Projects, especially in AI, are heavily time-bound, and if you feel that your team won’t be able to finish off the task within the given deadline, be it because of the established workflow or the project volume, you better outsource than fail.
Cost savings opportunity
From a cost-saving standpoint, outsourcing a data annotation project outbound is likely to profit your bottom line only if the quality provided corresponds to the agreed price.
Quite naturally, you don’t want to pay for poor results no matter the project urgency: your company reputation is put on scales. So, you should be careful with the company choice and research the service provider beforehand. We’ll discuss further precautions and key considerations when selecting a vendor later on in this blog post.
Implement a scalable solution
At this point, you might be wondering how does data annotation outsourcing contribute to a single comprehensive, scalable solution? The accumulation of all the previously discussed elements creates a surefire, value-packed outsourcing strategy that can not only cut you a nice chunk of effort but also give you enough time and available workforce to scale your CV pipeline exponentially.
Again, the role outsourced project annotation plays in a given CV model is highly dependent on your pipeline and individual features throughout your ML lifecycle.
Precautions when choosing the right vendor
Now that you’re familiar with the underlying benefits of outsourcing a data annotation project, let’s focus on key elements to consider when comparing the vendors. Here is the list of questions you should be asking to assist the selection:
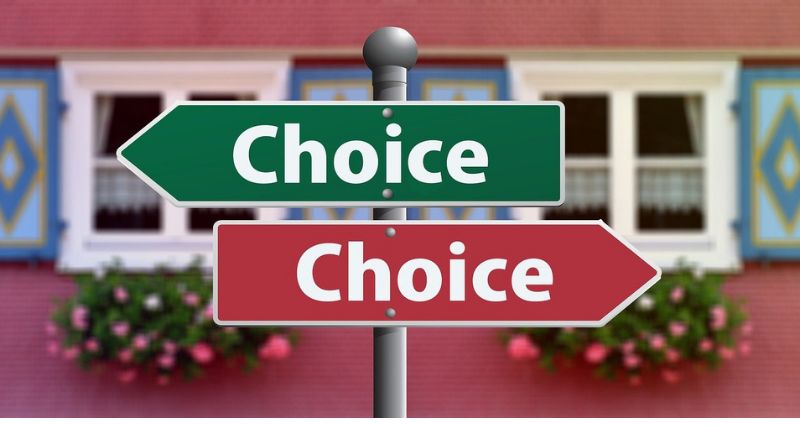
1) What type of data are you working with?
It’s likely that you will be choosing from a list of service providers specialized in a particular area, drone image annotation, or medical imaging, let’s say. In these events, your choice should be obvious.
2) What type of annotations needs to be implemented?
The tools the companies either offer or use may have limitations: some might be perfect for projects that require bounding boxes, others might provide robust tooling for semantic segmentation, and so on.
3) What is the main objective of data labeling service outsourcing?
Understanding the objective of outsourcing will also help you prioritize your project requirements accordingly.
4) Do you have a predetermined budget?
One of the most evident questions you should ask yourself is the budget. Or if the service costs above what you can pay, is it really worth it? Does it meet your project expectations?
5) How do you measure the efficiency of your project?
In other words, what are the KPIs for your annotation project, and are they precisely communicated to your service provider?
6) Is the selected annotation team provided with detailed instruction?
Understanding the requirements for digital annotation forsters quality results delivery, which also feeds your CV pipeline success.
Don’t be afraid to negotiate your package
This tip is an oldie but has proven effective over and over: shortlist key features for each data labeling platform and compare them one by one. Then negotiate with every service provider to see what additional functions they are ready to offer on top of what your package already contains. In the long run, you have nothing to lose; instead, you’ll understand the extent to which your vendor can be flexible to have you as a potential client.
Find your service provider match with SuperAnnotate
When in search of a professional vendor provider, make sure you don’t miss on opportunities by SuperAnnotate. SuperAnnotate is helping companies build the next generation of CV products with its end-to-end platform and integrated marketplace of managed annotation service teams. It was recognized as one of the world’s top 100 AI companies in 2021 by CB Insights.
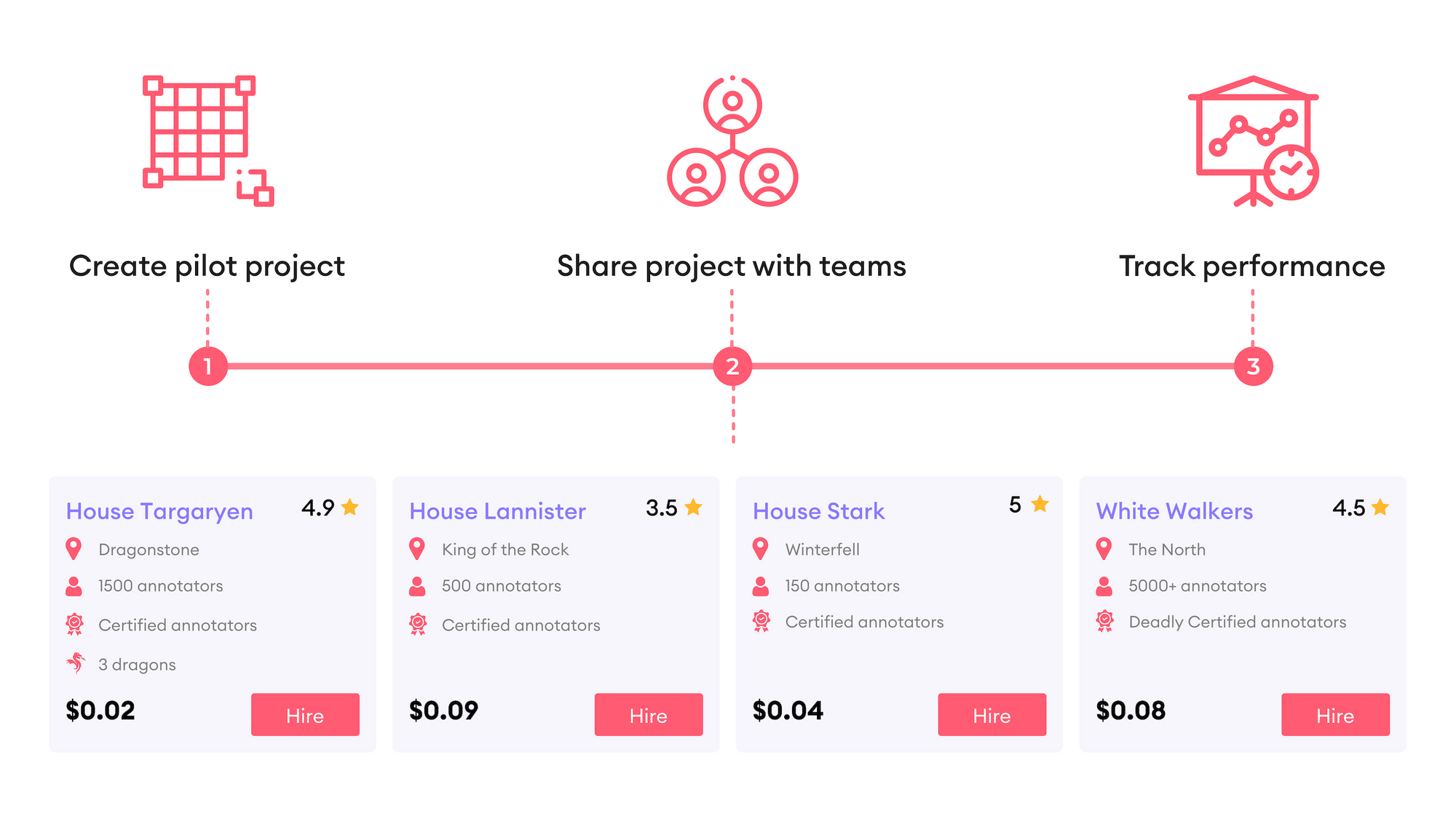
SuperAnnotate provides comprehensive annotation tooling, robust collaboration and quality management systems,no-code neural network training and automation, as well as a data review and curation system to successfully develop and scale CV projects. Everyone from researchers to startups to enterprises all over the world trust SuperAnnotate to build higher-quality training datasets up to 10x faster while significantly improving model performance.
Final thoughts
No matter how compelling in-house data annotation may seem, in most cases, it’s not the best decision for major projects, which explains why big companies tend to outsource data annotation projects so intensively.
As far as you’ve gotten to this section of the article, it’s likely that you’re considering data annotation outsourcing for your pipeline. If so, we recommend checking out our marketplace of highly equipped and trusted experts for customized deals.
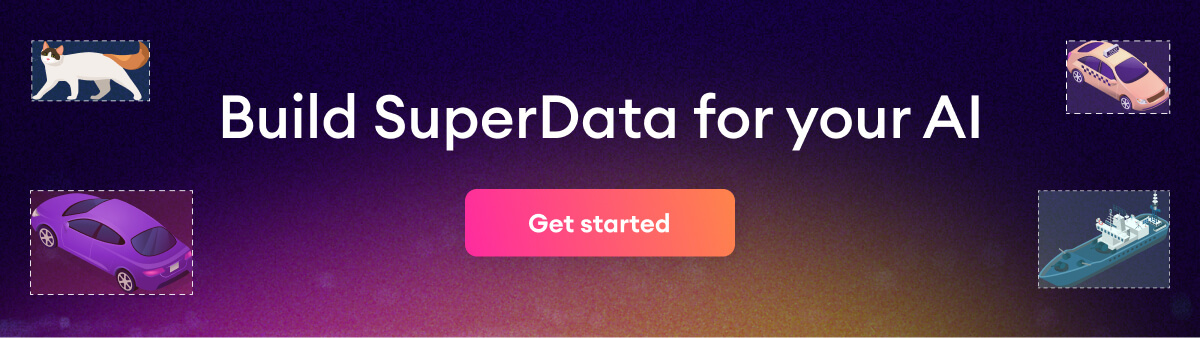