With the growth and spread of artificial intelligence (AI), other technologies have also been under development, one of which is data governance. According to the Data Management Association, the data governance definition entails the process of practicing control and authority over data assets management. Ultimately, data governance is all about finding the right balance between technology, process, and personnel to oversee the storage, input, and usage of data to attain administrative goals. In addition to delivering an enhanced quality of processed data, data governance best practices consist of ensuring the reliability of a data source, a full comprehension of customer needs, well-ordered data integration, respect for the regulations of the government, and a full-on advancement in data management while also serving to accommodate legal issues and security.

So buckle up, as we will be offering an overview of what data governance is, covering each feature on the data governance roadmap in the upcoming sections:
- Why is data governance important?
- Benefits of data governance
- Challenges in data governance
- Ethical implications and responsibilities of data governance
- Final thoughts
Why is data governance important?
Nowadays, all big businesses are competing with one another to attain the most operational business solutions through the usage of data analytics; they are extensively investing in AI-based solutions just so they can extract maximum worth from the data behemoth and improve their output.
Regardless of the significance of data quality improvement, other influential factors can contribute to ensuring an efficient decision-making process for a business. Some of the most prominent ones are trustworthiness and accuracy. In a sense, data governance and AI go hand in hand. As data governance enables the operation of AI through many means, its key contribution remains its simplification of high-quality data assembly.
As mentioned earlier, the data governance process can help businesses by picking out accurate data usage with regard to the rules and principles. But the real question remains, how? Data governance roles are pretty comprehensible, as they pertain to:
- Strategies
- High-quality data
- Trust increase
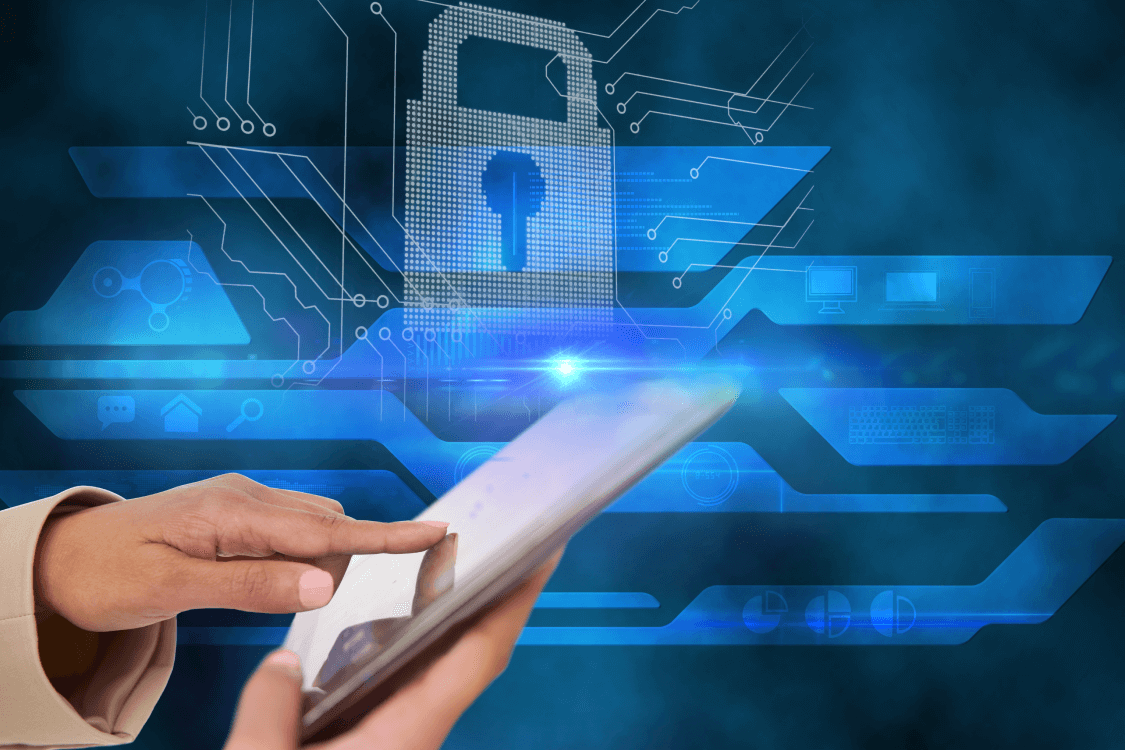
Strategies
Through proper implementation of a data governance strategy, a company can gain the following benefits:
- Trouble-free data assimilation: The data governance process uses a skill that allows organizations to sort out the location of any important entities’ data.
- Improved data quality: Data governance offers a standard that guarantees accurate data.
- Operative platform access: Data governance allows having an operative platform within the framework of government guidelines.
- Data management increase: Adding the human component to the data-driven environment. As data management creates fine practices and protocols, legality, security and compliance are tackled properly.
- All-around view for organizations: Through this, organizations can keep up with commercial entities and client needs.
High-quality data
Data governance contains a selection of actions that are aimed at managing data in a proper manner. An effective data governance framework highlights data quality by outlining its requirements and observing them against other related metrics. This role can be strengthened by data stewards’ identification to endorse responsibility and boost enterprise among shareholders so the data quality can match the necessary standards. Through this process, the need to maintain data quality will intensify, especially among those upstream of the data pipeline.
Trust increase
As helpful as they are, pleasant service offerings are never enough to attain a client’s trust, as they need to guarantee that their data is secure. This is when data governance gets involved, as the agreement of data laws in different parts of the world did alter the scope of data governance. Nowadays, organizations aspire to be identified with the data they process for directing their business.
That being said, data governance should be considered as an operational need rather than a set of rules. As the success of data management makes more room for improved insights, it can effectively enhance profits along with the purposes of any client or investor. The data governance operating model measures the consistency of a dataset by putting it up against a dependable reference dataset. This refers to one data field and it is commonly linked to the number of outliers that are triggered by sensor malfunctions, database failures, incorrect data assembly strategies, and much more.
Benefits of data governance
Data governance is essential, especially when dealing with massive datasets. Effective data governance frameworks allow businesses to regulate several business procedures and advance operative competence. Also, the emphasis on data quality through all stages of an organization allows data governance to enable data-driven analysis with or without the employment of AI.
Besides, data governance makes sure AI offers beneficial results not only because of its understanding of legal and ethical matters but also the essential implications that demonstrate what must be achieved.
Challenges in data governance
Just as any other technology, despite the innovative benefits of data governance, it does come with baggage:
- Framework adherence
- Legacy systems shift
- Rapid data growth
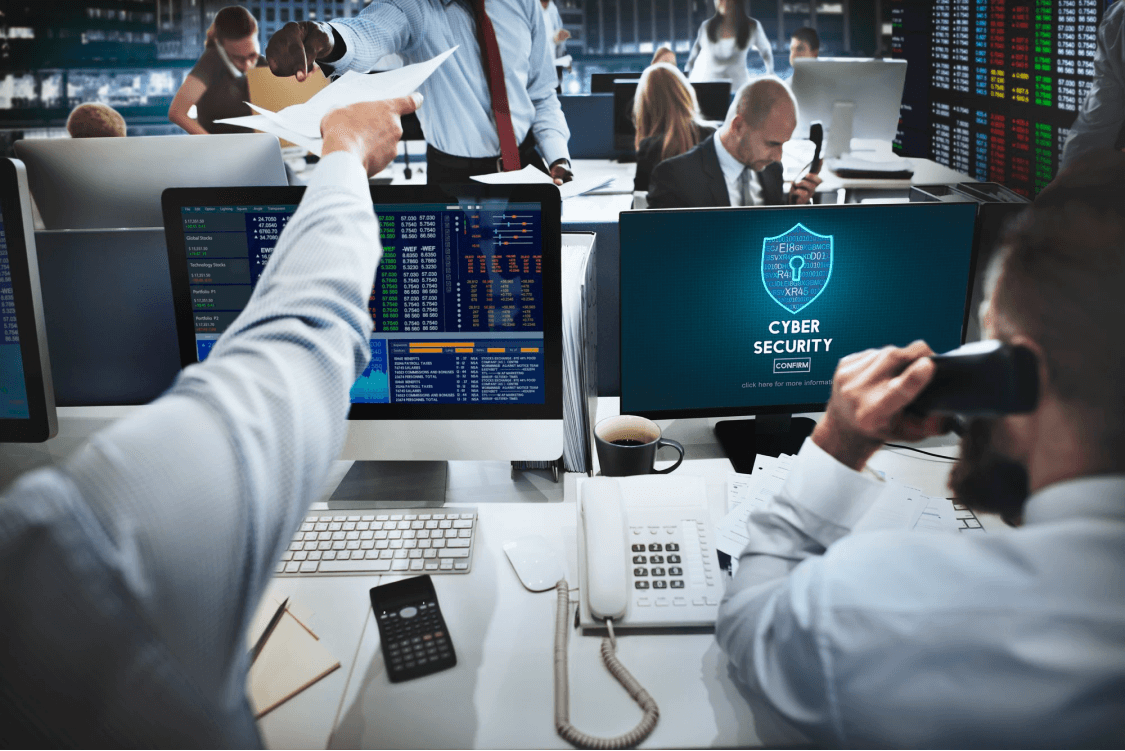
Framework adherence
Proper AI use is also connected to data source accessibility. The data should not be biased, and an organization’s data-related units should follow the organization’s framework so they can achieve proper data governance. When collecting and preparing training data for your AI, you can use SuperAnnotate’s Explore feature to effectively curate datasets, manage your data quality, and detect and mitigate/prevent biases before they are put into training.
Legacy systems shift
Old systems cannot carry on with the current data demands, and as a result, their presence is not required. As reasonable as this concept sounds to many, the immediate move to fresher systems can be more difficult than expected. In order to move on to newer systems, one has to come up with a framework that includes safety, scalability, and amenability that corresponds to the new system.
Rapid data growth
In the current AI era, the quantity of data production and storage has developed at a large scale, which indicates that computer technologies are thriving, and the number of devices that store data has also grown. With the growing number of devices, so is their use of the internet. Although these updates are embraced by most, the data expansion is affecting organizations and is leaving them in need of a trustworthy structure to maintain all this data.
Ethical implications and responsibilities of data governance
The General Data Protection Regulation and California Consumer Privacy Act are the modern regulation systems that are in charge of user data protection and the providence of standards that companies must pay attention to. If otherwise, they can get in trouble and receive serious penalties.

SuperAnnotate, for example, is committed to being the most trusted end-to-end platform to annotate, version, and manage ground truth data for AI as it complies with the following regulations: GDPR, SOC2, and HIPAA.
In many synchronized businesses, organizations still try to challenge data governance and AI ethics, which leads to the regulation of laws. By respecting AI governance policies and ethics, organizations set out the right message to the public, thus, instilling trust among the users.
To respect these regulations, an AI system should adhere to the following:
- Safe and trustworthy operation
- Respect for privacy and data protection
- Equal treatment for all of its users
- Algorithmic responsibility
Final thoughts
With all the benefits and challenges of data governance, it is as important to highlight how the guidelines for data governance will also need to develop with the emerging laws, adaptations of new technologies, and business practices. Also, it is as important for organizations to reconsider the ways they will be using data in regard to processing and storing. By now, it is no secret that AI presence has a positive effect, and with the existence of automation in organizations, the implementation of security and obedience in their data centers can be improved. It goes without saying that the true essence of data is in the support it provides to the decision-making processes of a company. Yet any company that is considering investing in AI should also have governance of AI (i.e., data governance framework). Through this, they will guarantee data quality, which can lead to greater opportunities and earn a better reputation in today’s highly competitive market.
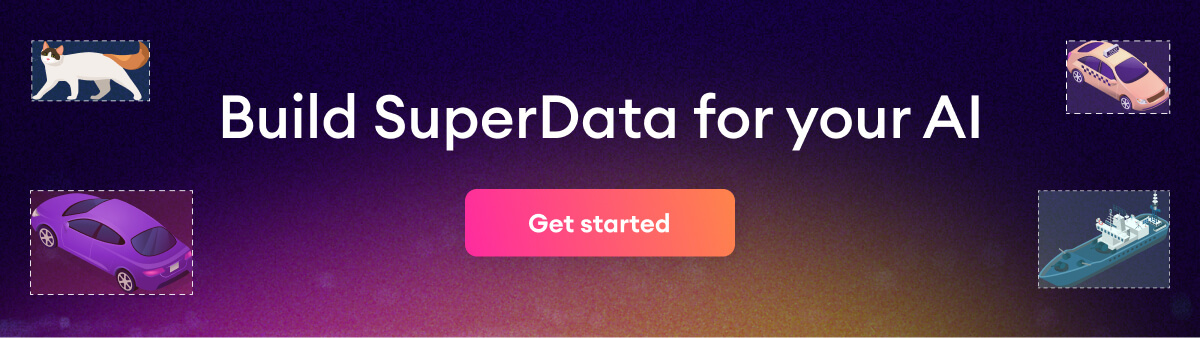