Artificial intelligence (AI), machine learning (ML), and computer vision (CV) are indeed changing the way we live, impacting a number of industries, such as autonomous driving, agriculture, healthcare, and many more. Insurance is not an exception; as one of the key fields where we work and play, it is inevitably subject to technological advancements.
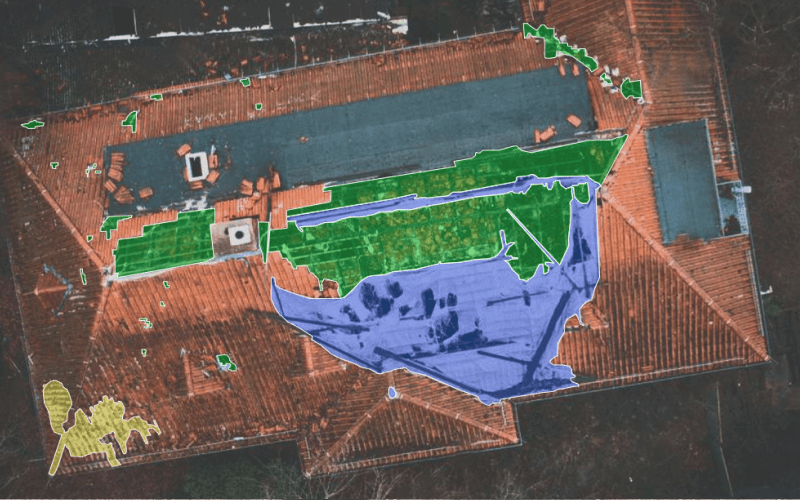
The insurance industry is leveraging emerging artificial intelligence technologies, pushing the demand for digitization even further. With deep learning vision systems, computer vision enables computers to "see" and "understand" objects and events with an accuracy level that sometimes surpasses humans. That's why engineers and experts from the insurance industry develop real-life computer vision applications at an accelerated pace. Below, we'll introduce the top six use cases of how computer vision brings about innovative solutions to the insurance field.
- Fraud detection
- Vehicle damage assessment
- Roof underwriting
- Wildfire risk assessment
- Surveillance on construction sites
- NLP for insurance
- Key takeaways
Fraud detection
Insurance claim fraud has always been a major problem in the industry. On the one hand, there's the risk of affecting client satisfaction by delaying payouts or conducting lengthy investigations at a stressful moment. Besides, there are investigation costs and regulatory pressure from the insurance business. On the other hand, incorrect payouts reduce profitability and encourage other policyholders to engage in similar delinquent behavior.
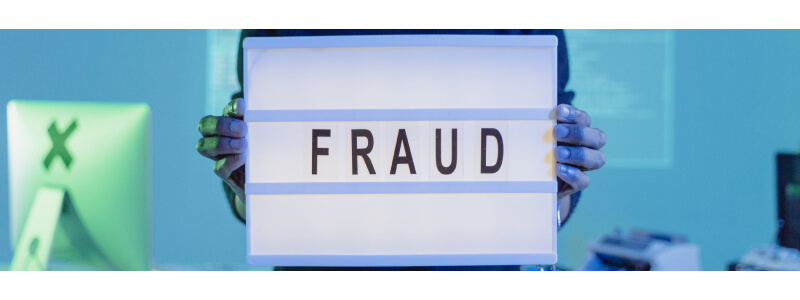
With various fraud types and the low ratio of known frauds in typical samples, detecting insurance fraud is a difficult task. When developing fraud detection models, we must balance the costs of false warnings against loss avoidance. Machine learning and computer vision foster fraud prediction algorithms based on previous experience. Artificial intelligence fraud detection applications can even be used to do background checks for new customers, carefully calculating the risks linked to businesses and people. For instance, a computer vision model can identify particular elements, such as invalid documents, fake images, and alert once such have been detected. On top of that, NLP technologies can analyze unstructured data such as messages, claims, and customer feedback and then notify humans about incidents of suspected fraud. In addition, recent computer vision methods improve forecast accuracy, allowing loss control units to cover more cases with fewer false positives.
Vehicle damage assessment
When a vehicle crashes in an accident, the liable party applies for insurance and goes through a collision damage assessment. The primary purpose is to evaluate the required repair work and the amount of reimbursement. This is a manual task that takes a lot of time and human resources: the assessor should methodically and accurately estimate all aspects of damage to determine which vehicle elements should be fixed or replaced based on information provided by manufacturers. These datasets provide a more accurate assessment with machine learning algorithms compared to the human eye. Due to computer vision, it is possible to locate the damage and analyze its extent to a vehicle within seconds. When you add computer vision insurance to the equation, it automates assessors' work and helps quote the just claim amount to resolve the issue. If building such an AI model is something you're looking into, note that data quality is a crucial component for successful implementation.
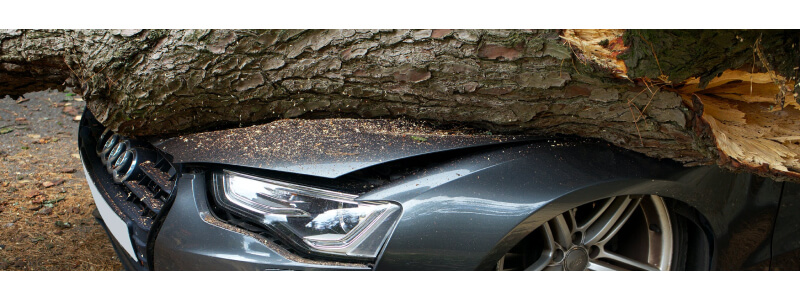
Roof underwriting
A roof is one of the most essential aspects of a property from the standpoint of underwriting. Unfortunately, validating the roof age has always been a challenge for the insurance industry. Insurers have traditionally relied on information supplied by the homeowner or agent, an inspector's visual assessment, or the house's year of construction. These standard procedures can miscalculate the roof's actual age, and the result costs insurers an estimated $1.14 billion in yearly premium leakage. In addition, property change events, and severe natural conditions such as winds and thunderstorms further complexify the underwriting workflows.
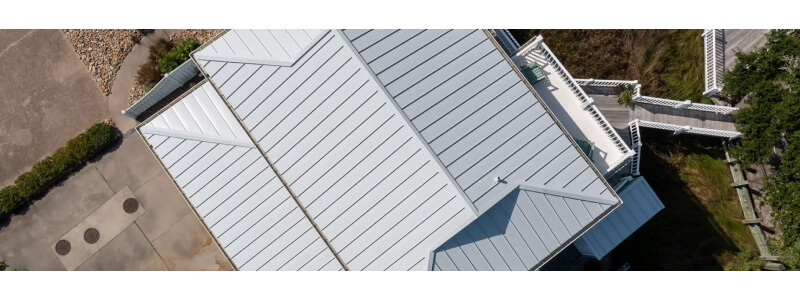
Computer vision provides those who are in the property insurance sector with data to verify the age, condition, and characteristics of a roof, as well as its potential for hail and wind damage. This supports insurers in two ways, helping accelerate underwriting and assess future risk with higher accuracy. With high-resolution views and aerial imagery, it is possible to monitor how the roof condition changes over time and capture potential hazards, such as nearby or overhanging trees, materials that are especially prone to damage, and expensive attachments, such as solar panels.
Wildfire risk assessment
Given the ongoing expansion of houses and businesses in wildland-urban regions, wildfires are becoming a significant cause of catastrophic destruction and insurance losses.
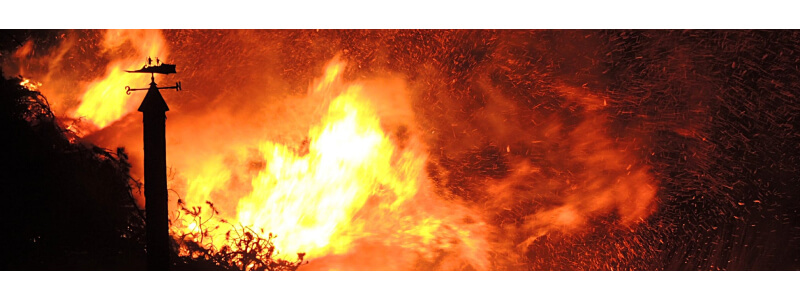
As the wildfire threat grows, computer vision helps us monitor property-level mitigation using aerial imagery to measure property elements like elevation and acreage, as well as monitor characteristics like defensible space between structures and vegetation or other potentially combustible materials for wildfire mitigation.
It is feasible to create property-specific hazard scores based on fuel, slope, and access risk elements and detect dangers exposed to wind-borne embers using computer vision. Community-level mitigation information and historical wildfire activity are two further areas of investigation for computer vision.
Surveillance on construction sites
Once a home is built and purchased, the owners get a homeowner's insurance policy or take out other types of property insurance to safeguard their investments. There's also the builder's risk insurance – it covers a building or property that is presently under construction. This type of coverage often protects both the structure and the on-site construction materials. Employees of the construction company are also protected by insurance in an accident.
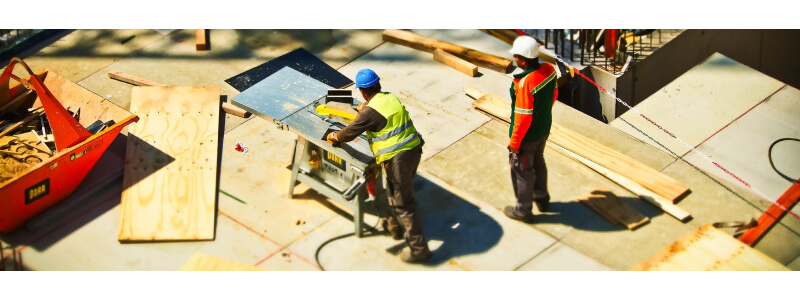
Computer vision surveillance on construction sites aims to minimize unfortunate occurrences and ensure a safe environment for the employees. The implementation of the latter requires detailed information on the following:
- If eligible employees are using the necessary equipment
- If cases of accidents are covered
- If processes are executed in a prescribed way
- If there are signs of failure that would cause insured damage to the site and more.
Luckily, a computer vision-powered surveillance system covers all of the above in real-time.
NLP for insurance
It's no surprise that insurance companies are associated with tons of paperwork and bureaucracy. Insurance agents spend a considerable amount of their time manually filling in or transferring information. As 80% of data in today's insurance companies are texts, natural language processing (NLP) can be actively implemented in the field. You may ask, what is NLP exactly? In short, it's a subfield of artificial intelligence that enables computers to automatically read, understand, and derive meaning from text in a variety of contexts. In the insurance industry, this is typically done by having the technology analyze a large number of claims in order to create a database of knowledge, automate data entry, data validation, and even fraud detection. Instead of spending hours manually re-writing the documents, people who work in insurance can use automated systems to factually capture data from the papers and documents. Here are a couple of more detailed examples of NLP applications in insurance.

Claims management
Claim management is one of the critical processes for any insurance business that involves claim registration, damage estimation, determining which party is liable for the damage, paying for the damage, reporting back to the client, and so on. Insurance organizations are under constant pressure to offer improved services at reduced costs — particularly for claims management. It goes without saying that this is a lot of paperwork that requires thorough attention to avoid errors and prevent fraud. With advanced machine learning techniques, businesses can harness non-traditional data to automate claim management, detect incremental fraud patterns as well as minimize false positives that allow differentiating between genuine and fake data points.
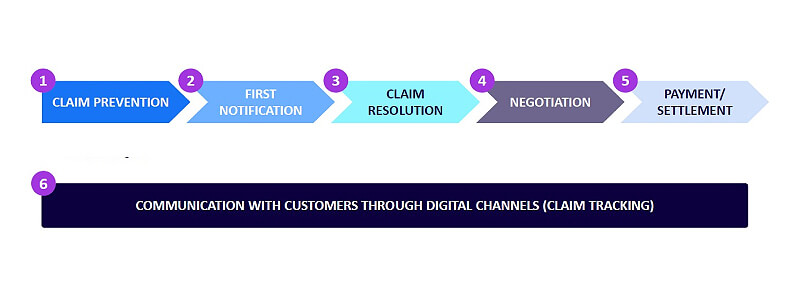
Customer support
When surfing the net, you may have encountered a chatbot that answers your questions or offers you to view specific (relevant) items. These virtual assistants powered by NLP technologies have been around for some time now. NLP-trained bots help deliver a personalized experience to insurance customers as in any other field. Such a solution empowers insurance businesses to tackle more inquiries in a notably shorter amount of time and save the human resource for more high-value tasks. Besides answering questions on their own, NLP-powered models can assist customer support employees in doing research, and quickly filtering, finding, and classifying existing claims.
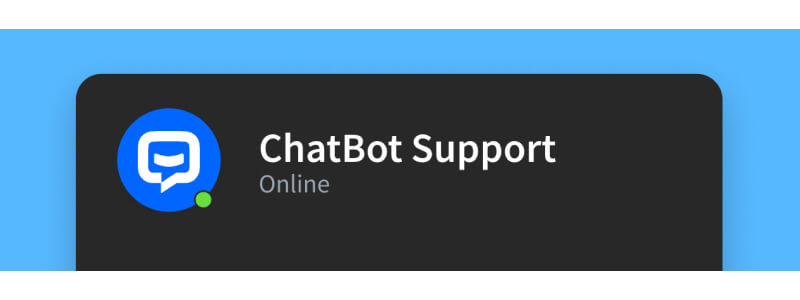
Key takeaways
Nowadays, computer vision contributes to nearly every aspect of our lives. It has proven to be beneficial in diverse areas, including security, surveillance, healthcare, agriculture, finance, and many more. Insurance companies profit immensely from computer vision since it aids in the automation of lengthy operations, including time-consuming paperwork. Computer vision-based technologies reduce the time it takes for consumers to get their reimbursements, enhance risk management, and help insurers minimize claims leakage, saving money. When creating a computer vision model, it's critical to remember the one and only factor that defines the future model's performance: data. It would be impossible to achieve accurate predictions without clear and consistent data, let alone cover edge cases, which might have major ramifications in the insurance industry.
SuperAnnotate makes it easier for companies like yours to annotate, label, and utilize higher-quality data. If you'd like to learn more about how SuperAnnotate may benefit your company, schedule a call, and we'll be happy to answer your questions.
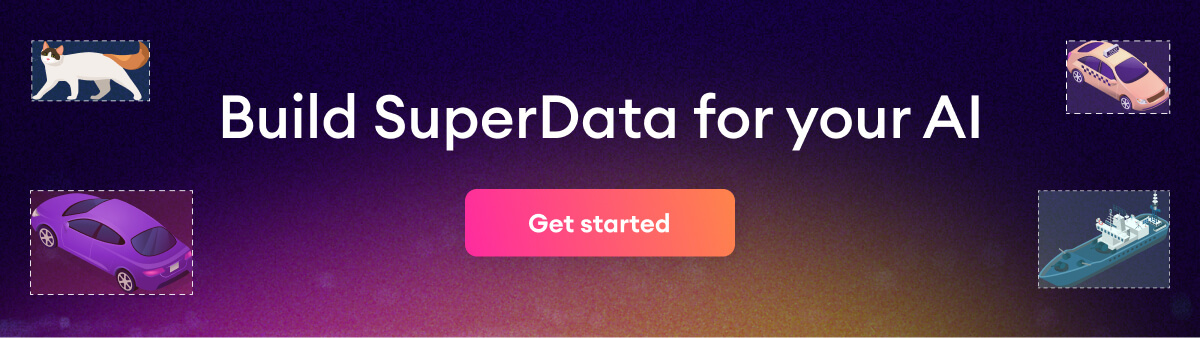