In this day and age, when there is a technological solution to almost everything, wildfires continue to swell intensively, posing a real threat to wildlife. About 3.6 million acres were burned in the US only throughout 6 months in 2021. One of the ways to combat these fires is immediate firefighting, which not only requires substantial human and financial resources but is also a dangerous activity causing a great number of casualties each year. While these fires are a natural part of the ecosystem, their abundance and sheer lack of control can bring about catastrophic environmental, social, and economic impacts. Traditional methods of wildfire detection such as watchtowers are no more efficient, so real-time forest fire perception is one of the key objectives to develop sustainable and effective fighting strategies. Unmanned aerial vehicles (UAV) have also been used extensively in the last few years to assist in monitoring procedures and fire detection. Either way, the use of computer vision (CV) can contribute a great deal to work out techniques to safeguard forests through measurement and visualization of fire features. Moving on, we will address the following in this blog post:
- Smoke vs. fire detection
- Fighting from space
- Why use UAVs for fire detection
- Challenges and constraints in CV for wildfire detection
- Current efforts
- Key takeaways

Smoke vs. fire detection
State-of-the-art image recognition owes much of its success to deep convolutional neural networks. Yet, these are not often implemented for smoke and fire recognition, so consequently, the constraints of this particular use case are not thoroughly discussed. Before going into the specifics of how CV fosters fire detection and prevention, let’s set one thing clear right at the outset: object detectors based on fire detection methods can be grouped into two classes: flame and fire detection. Quite commonly, the smoke generated by these fires is detected much earlier than the flames. So, smoke detection acquires more attention in this context, as it also impacts fire alarms and can contribute to preventing fires before they expand massively. As a rule of thumb, smoke detection is the starting point of fire detection.
Fighting from space
The reality of firefighting is not as romantic as this subtitle. Most fires nowadays are reported either through emergency calls, commercial flights, or lookout stations, which is already a limitation. The sad truth is that some wildfires are determined when acres are far beyond burned, whereas others remain completely undiscovered. One way to address this problem is satellite-focused imagery in exhaustive detail to spot thermal activity. As expected, NASA has already had its own contribution to this: two NASA satellites went into use to orbit the earth and scan it for excessive fire activity. James MacKinnon, a computer engineer at NASA Goddard Space Flight Center, worked on developing a neural network on a year’s worth of satellite images that achieved 98 % accuracy in wildfire detection. The end result is promising, yet that was only one of the applications of CV in satellites that fosters effective disaster management. Today CV serves as the outer orbit’s eye to track the activity on earth and derive insights. It is also embedded into a number of other government applications other than wildfires, including:
- Agricultural monitoring to identify and measure the main crop production areas.
- Humanitarian aid to alleviate human suffering.
- Animal migration tracking for long-distance movement of animals.
- Climate change: big time!
- Fisheries management to prevent overfishing.
- Weather forecasting, to analyze data on the changes in climate and make predictions.
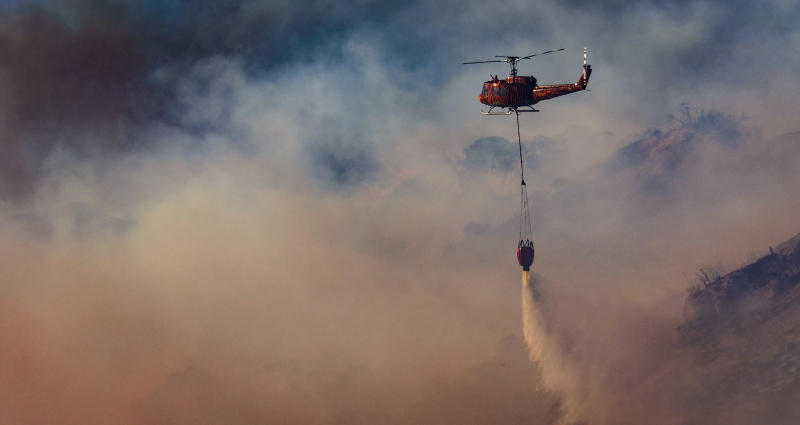
Why use UAVs for fire detection
UAVs have demonstrated tremendous progress in the last decades and have proven useful in multiple areas: wildfires are no exception. Because of their high maneuverability and remote sensing, UAVs serve best for task planning and allocation strategies, providing a budget alternative to detecting and preventing wildfires. Remote sensing specifically has a handful of advantages for emergency assistance, allowing for a thorough inspection of a given area and autonomous navigation across the defined path. UAV sensors capture target data to monitor the scene and provide emergency response as necessary. GNSS and INS specifically are great for UAV localization, allowing for geographical mapping of fires. At this point, it is crucial to consider fire features or elements such as heat, motion, brightness, flickering, smoke, etc., which again are measured by sensing devices. A common example of a sensing device is a camera with its own variety of gauging metrics.
Back to the initial question: why UAVs at all? Manually controlled UAVs reduce the risk for humans and minimize their intervention in life-threatening tasks. Besides, the automation of maneuvers, planning, and other mission-related tasks through a CV improves distant surveillance and monitoring, thus, streamlining the process of fire detection in the long run. Long story short, the evolution of UAVs directly influences firefighting resource management and disaster mitigation.
Challenges and constraints in CV for wildfire detection
Just like for every CV project, here too, there is an entire list of challenges and limitations to consider before developing an algorithm that can detect wildfires. We’ll go through several of them:
Scarcity in data
The first major constraint in wildfire detection is data scarcity. Open-source datasets do not necessarily cover a vast quantity of wildfire images. So, if you are about to take over a project that is aimed at detecting forest fires through CV, make sure you have sufficient data. By saying data, do not limit yourself with images only. Wildfires are one of those areas where video annotations may serve your project purposes better, as wildfires start off small and develop to a critical point. The process of that development is best expressed through footage, as opposed to static images, and that footage has to be labeled, which assumes a fair amount of human and financial resource investment.
Difficulty in feature-tracking
And just like that, wildfires have one of the most complicated features to obtain through CV, including flame height, flame inclination angle, firebase width, etc. Any deviation or improper prediction of the measures above can result in poor model performance.
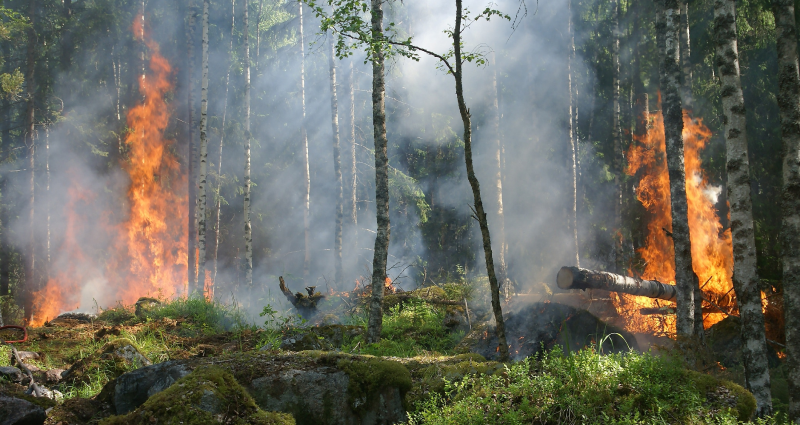
Uncontrollable or sudden changes in the environment
Another restriction, a common one across CV-integrated industries, is the external influence. Especially in forests or fauna, we don’t have full control over the environment. Whether it’s animal migration to the target area or an unexpected disaster in parallel with wildfires, the environmental conditions the model is trained on may not always overlap with the actual settings. Calibration problems are almost unavoidable, as you saw.
Predictive fires are only the minority of the existing fires
With the use of CV for fires, there comes the concern of probability. AI machines trained on data provided by a human can only detect predictive fires, their severity, and trajectory, but nature is not that simple. In fact, not even close to what we expect, considering that the detectable fires are only 10% of the actual wildfires all around the world. As luck would have it, fire behavior in urban and especially wildlife environments does not have enough predictability yet. Even though we do not have the capacity to estimate the exact probability of wildfires in a given area, we still track recurring patterns and draw conclusions. The predictive analytics based on these insights becomes more and more efficient in fire detection, mitigation, and prevention. So, the control we have over real-time fires is refined day by day. All is left is to focus on studying non-predictive fires to be able to train models for them too.

Current efforts
The only widely implemented instantaneous response mechanism available as of now is firefighting, which, let’s admit, doesn’t outsmart fires but only delays or mitigates their effects. Another common effort is building fire-resistant residencies and houses: a short-term solution, much like firefighting. However, the transition to smarter CV-inspired alternatives is not miles away. Although not massively implemented, individual research centers and engineers do make efforts to gradually shift to CV fire detection solutions: fire mapping guided by weather prediction is only the beginning.
Key takeaways
Fair enough, wildfires is a continuously burning topic. Of course, there are benefits to wildfires as well, such as recycling all the dead stuff into forests. Yet, we can be more strategic about safeguarding forests and people by having a little more authority over the disastrous side of it. CV integration, in that sense, is on our priority list. We hope this article provided you with a general understanding of how CV can detect and prevent forest fires. Thought of developing your own fire detection model and need annotated data? Our marketplace of annotation services may come in handy. Let us know if you have questions or need further information.